Gaining insight into where earthquake aftershocks might occur is a long-standing challenge in earthquake science, especially as some aftershocks can approach the size of the mainshock earthquake and can damage buildings and roads already weakened by the main earthquake. Phoebe Robinson DeVries and her coauthors are pursuing one way to strengthen aftershock location forecasts—using a type of artificial intelligence called deep learning.
Deep learning programs use hierarchical, multiple layers of data processing to find patterns within a large, complex set of information, with each successive layer of data acting as a slightly more abstract representation of the original data. Deep learning approaches are already used in computer programs that recognize natural language, like Siri and other smartphone digital assistants, classifying and identification of images “and all sorts of other pattern recognition and perceptual problems,” says DeVries.
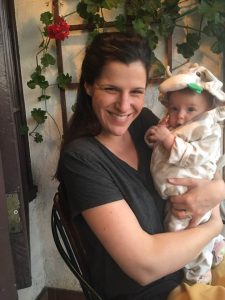
The backbone of these programs are neural networks—a set of algorithms that underpin deep learning. “One way to think of neural networks is as ‘function finders’–algorithms that can find extremely complex and non-linear relationships between inputs and outputs,” she explains.
This summer, DeVries, who is completing an earth and planetary sciences postdoc at Harvard University, and her colleagues published a paper on a deep learning program that looked for patterns in the complex relationship between earthquake mainshocks and aftershocks. The program was “trained” on data from more than 100 mainshock earthquakes, including the magnitude 9.1 Tohoku earthquake that devastated Japan in 2011, and 131,000 aftershock earthquakes. Based on modeled stress changes around the mainshock earthquakes, the neural networks were trained to forecast aftershock locations without any prior assumptions about the directions of the other faults in the area.
When the researchers tested the program against another 30,000 mainshock-aftershock pairs, they found that the program did a better job of explaining aftershock locations than a commonly-used method that looks at how stress changes in rocks around an earthquake may lead to an aftershock in a specific area.
It was Harvard professor Brendan Meade, DeVries’ Ph.D. and postdoc advisor, who sparked the researchers’ interest in combining earthquake studies and artificial intelligence. When Meade came back to his Harvard research group after a sabbatical at Google, “he got us thinking about and brainstorming applications in earth science [for deep learning approaches.],” DeVries says.
Deep learning approaches offer a new way to dig deeper into earth science data, by letting the data analysis itself guide the discovery of unexpected patterns and relationships in earthquake dynamics. “Instead of starting with any particular theory or hypothesis, neural networks can be used to find empirical relationships between physical phenomena and observations,” says DeVries.
Programming was a natural step for DeVries’ career, after an early introduction to computing. “My parents sent my sister and me to computer camp every summer when we were in middle school. It was much more fun than we expected! We learned to program in BASIC and played computer games. Eventually in college I learned the basics of MATLAB, C, and Python through research projects and classes, but always appreciated the early and positive introduction to programming in computer camp,” she says.
Although she had wide interests in science, politics and history in college, DeVries focused on applied mathematics “because it seemed like a major that would give me skills that could be useful for a lot of different areas.”
Teaching is another passion for DeVries, now an assistant professor in the physics department at the University of Connecticut. While at Harvard, she worked as a teaching fellow for Harvard’s Natural Disasters and Introductory Geology courses and a resident tutor and academic advisor for Harvard College sophomores.
“I love teaching,” she says. “Research is rewarding in the long term, but to me teaching and advising is rewarding on a shorter time scale.”
The March 2019 issue of Seismological Research Letters will contain a focus section on deep learning and other machine learning approaches to seismology
SSA At Work is a monthly column that follows the careers of SSA members. For the full list of issues, head to our At Work page.